Genetic Algorithms: Difference between revisions
Mr. MacKenty (talk | contribs) No edit summary |
Mr. MacKenty (talk | contribs) No edit summary |
||
Line 7: | Line 7: | ||
== A video to get you started == | == A video to get you started == | ||
<html> | |||
<iframe width="560" height="315" src="https://www.youtube.com/embed/uQj5UNhCPuo" title="YouTube video player" frameborder="0" allow="accelerometer; autoplay; clipboard-write; encrypted-media; gyroscope; picture-in-picture" allowfullscreen></iframe> | |||
</html> | |||
<br /> | |||
<br /> | |||
<html> | <html> |
Revision as of 13:30, 1 December 2021
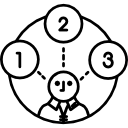
Advanced programming[1]
In computer science and operations research, a genetic algorithm (GA) is a metaheuristic inspired by the process of natural selection that belongs to the larger class of evolutionary algorithms (EA). Genetic algorithms are commonly used to generate high-quality solutions to optimization and search problems by relying on biologically inspired operators such as mutation, crossover and selection[2]
- Please first review the characteristics of algorithms.
- Please then review the characteristics of heuristics.
A video to get you started
Start here to understand genetic algorithms
- Brute force approach
- Combinatorial optimization
- Computational intractability
- Convergence
- Crossover / crossover operator
- Elitism
- Exploration vs exploitation
- Fitness / fitness function / fitness landscape
- Heuristics
- Hill climbing
- Initialization parameters
- Local extrema
- Mating pool
- Mutation / mutation rate
- Novelty search
- Offspring
- Optimization
- Population
- Premature convergence
- Problem space
- Ranking
- Roulette wheel selection
- Selection strategy
- Simulated annealing
- Stochastic universal sampling
- Termination condition
- Tour
- Tournament selection
- Truncation selection