Semantic Web: Difference between revisions
Mr. MacKenty (talk | contribs) No edit summary |
Mr. MacKenty (talk | contribs) |
||
Line 17: | Line 17: | ||
The goal of the Semantic Web is to associate meaning with the data on the Web and to exploit the wealth of data on the Web through more intelligent (meaningful) processing. A semantic approach to data processing, such as the use of ontologies or knowledge bases, has increasingly been integrated with other AI techniques, especially machine learning (ML) and natural language processing (NLP).<ref>https://www.quora.com/What-is-the-main-goal-of-semantic-web</ref> | The goal of the Semantic Web is to associate meaning with the data on the Web and to exploit the wealth of data on the Web through more intelligent (meaningful) processing. A semantic approach to data processing, such as the use of ontologies or knowledge bases, has increasingly been integrated with other AI techniques, especially machine learning (ML) and natural language processing (NLP).<ref>https://www.quora.com/What-is-the-main-goal-of-semantic-web</ref> | ||
* Examples of non-semantic elements: <div> and <span> - Tells nothing about its content.<ref>https://www.w3schools.com/html/html5_semantic_elements.asp</ref> | |||
* Examples of semantic elements: <form>, <table>, and <article> - Clearly defines its content.<ref>https://www.w3schools.com/html/html5_semantic_elements.asp</ref> | |||
Revision as of 18:39, 22 January 2018
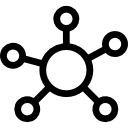
Web Science[1]
Text web vs multimedia web[edit]
Text Web | Multimedia web |
---|---|
Text is presented with minimal markup | Text is heavily styled often with CSS |
Pages are generally static (dynamic elements are certainly feasible, but they are often minimally styled) | Pages are generally dynamic |
Page is designed to be read | Page is designed to be seen, heard, and read |
Design of content and markup is often rather sparse and utilitarian | Design of content and markup is often called "rich media". |
The aims of the semantic web[edit]
The goal of the Semantic Web is to associate meaning with the data on the Web and to exploit the wealth of data on the Web through more intelligent (meaningful) processing. A semantic approach to data processing, such as the use of ontologies or knowledge bases, has increasingly been integrated with other AI techniques, especially machine learning (ML) and natural language processing (NLP).[2]
- Examples of non-semantic elements: and - Tells nothing about its content.[3]
- Examples of semantic elements: <form>, , and <article> - Clearly defines its content.[4]
From the W3C[5]:
- The Semantic Web is an extension of the current Web that will allow you to find, share, and combine information more easily.
- Designed to be a universal medium for the exchange of data
- Enable vocabulary semantics to be defined and reused by communities of expertise, not necessarily W3C
- Provide for the fine-grained mixing of diverse metadata
- Making it cost-effective for people to effectively record their knowledge.
Do you understand this?[edit]
From the IB: The traditional web is seen as being text based, the semantic web is multimedia based.
Standards[edit]
These standards are used from the IB Computer Science Subject Guide[6]
- Distinguish between the text-web and the multimedia-web
- Describe the aims of the semantic web
References[edit]
- ↑ http://www.flaticon.com/
- ↑ https://www.quora.com/What-is-the-main-goal-of-semantic-web
- ↑ https://www.w3schools.com/html/html5_semantic_elements.asp
- ↑ https://www.w3schools.com/html/html5_semantic_elements.asp
- ↑ https://www.w3.org/2003/Talks/0522-swa-em/slide3-0.html
- ↑ IB Diploma Programme Computer science guide (first examinations 2014). Cardiff, Wales, United Kingdom: International Baccalaureate Organization. January 2012.